Plymouth, 26. June 2007
Meet the Cube-Tec Team at IAFPA 2007 Plymouth
The 2007 IAFPA Annual Conference will be hosted by the College of St Mark and St John, Plymouth, UK. The conference will take place from Sunday 22nd July 2007 (reception and registration) until Wednesday 25th July 2007 in the college's Desmond Tutu Centre. IAFPA

Meet Tom Lorenz and Joerg Houpert in Plymouth, Mr Houpert will give a talk about the results of a joint reseach project:
Increasing Speech Intelligibility by DeNoising: What can be achieved?
Jan Rademacher:
Medical Physics – Signal Processing Group, University of Oldenburg, Oldenburg, Germany
Prof. Dr. Joerg Bitzer:
Institute for Hearing Technology and Audiology, University of Applied Science Oldenburg / Ostfriesland / Wilhelmshaven, Germany
Joerg Houpert:
Cube-Tec International GmbH, Anne-Conway Str. 1, 28359 Bremen Germany
Abstract:
A lot of forensic material is of poor recording quality. A variety of disturbances is added to the desired speech signal. A typical way to increase the quality of the signal is to apply algorithms that can be called DeNoisers, which filter out the background noises. However, increasing the quality does not imply that the speech intelligibility is increased, which is the essential goal of the process.
In (Bitzer et al. 2005) the authors show that no significant enhancement is possible if the background noise is a perfect masker for the desired speech signal. In their study the noise signal was computed by a mixture of 500 sentences randomly mixed by the same speaker. Therefore, the long-term noise spectrum is exactly the same as the spectrum of the desired speech signal. Finally, the speech reception threshold is computed, which is the Signal-to-Noise-Ratio (SNR) at which 50% of all words can be understood. The methodology is described in (Kollmeier and Wesselkamp 1997 and Wagener 2003). The test is called Oldenburger sentence test and is based on (Hagerman 1982).
In real world recordings, however, the masker has a different spectrum. Prediction of enhancement The differences of the long term spectrum of the desired signal and the disturbance can be used as a measure of how well a denoiser could work for this combination of signals. Of course this is a coarse prediction since stationarity has to be assumed. Our new measure is based on the so called “optimal-” or “Wiener- filter” (Boll 1979, Wiener 1949) which is well-known for denoising tasks.
Based on this filter, we suggest to calculate the masking thresholds (MT) (Zwicker 1982) in a way closely related to the MPEG standard (ISO/IEC 11172 1993) both for the original noise spectrum and for the noise spectrum denoised by the Wiener-filter. These MTs are then used to find the spectral components of the speech signal which are audible before and after the denoising process. Taking into account the “band importance function” (American National Standard 1997) reflecting the importance of each frequency band for intelligibility, the increase of audible frequency bands after denoising will be a good approximation of the forthcoming improvement of the intelligibility achieved by denoising.
In order to show this behaviour subjective listening tests will be used for comparison. Conclusions: Increasing speech intelligibility is possible, if the disturbing signal is not masking all important frequencies of the desired signal. Since denoising algorithms are able to filter signal-bands individually a significant improvement in quality and intelligibility can be achieved. Furthermore, we introduced a new measure that can be used as a coarse predictor of the possible improvement.
Maybe someone will remember, Mira Wedemeyer's presentation 3 years ago at the 13th IAFPA/IAFP Annual Conferences - 2004 - Helsinki, Finland
Automatic Speaker Identification in Forensic Analysis; How Useful Is It?
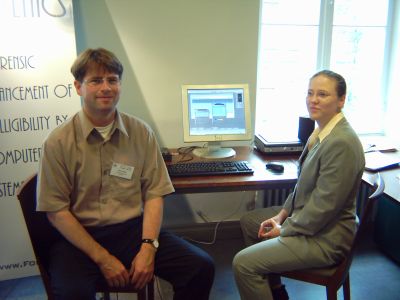
Abstract:
A problem often encountered in connection with forensic investigations is the identification of a criminal or witness by an excerpt of his voice. Naturally, it would be useful to have an automatic speaker identification via computer. During the last years, more and more research has been done on this topic, as there are many useful applications for speaker identification aside from forensic analysis, for example the recognition of a news anchorman for an automatic metadata extraction. Speaker identification is split into two main challenges.
The first challenge is the extraction of features that may represent the speaker. Commonly used features are the Mel Frequency Cepstral Coefficents (MFCC’s), and the Audio Spectrum Envelope (ASE), which is defined in the MPEG-7 standards. Both extraction methods work in the frequency domain, and are based on the acoustical properties of the human ear. Apart from these methods, delta coefficients are often used to characterize a speaker. These coefficients represent the temporal changes of the above-mentioned features.
The second challenge in speaker identification is assigning the features to a speaker. This challenge is encountered by methods such as Vector Quantisation (VQ), or Gaussian Mixture Model classification (GMM). In this contribution the aforementioned methods for speaker identification are introduced.
Following this, the benefits of these methods for forensic analysis are investigated. In forensic analysis, speech signals are often highly distorted so that automatic speaker identification may fail. The causes of these failures are shown and a final evaluation of the benefits of speaker identification in forensic analysis is given.
12th IAFPA/IAFP Annual Conferences - 2003 - Vienna, Austria
Detection, Interpolation and Cancellation Algorithms for GSM burst Removal for Forensic Audio Joerg Bitzer and Jan Rademacher
Abstract:
One increasing problem for forensic audio analysis is the so-called 'bumblebee noise', caused by GSM radio transmission. The disturbance can be described as short pulses with a fundamental frequency of approximately 217Hz. The pulse nature of the signal causes a lot of harmonics and therefore, most of the desired speech signal is masked. In this contribution we introduce a new algorithm to reduce the burst. After analysing the burst structure we found that filtering or noise cancelling is not suitable for this problem, because of two reasons. The burst itself is time-varying, or at least the recording device and medium are not constant and the burst distorts the signal more or less completely (overload, clipping). Therefore, we propose a detection and interpolation approach. Due to the large variety of occurrences over different recordings (inter-variation) and the small variations for one recording (intra-variation) we decided to use a fingerprint approach for detection. This means, the user selects one typical burst and the algorithm will find all other occurrences. This part of the signal is finally removed and interpolated by using model-based interpolation algorithms. The resulting speech signal sounds natural and the remaining noise is not disturbing or reduces intelligibility.